5-Out: Using Machine Learning to Transform Restaurant Operations
Branden, the co-founder of 5-Out, provides wonderful insights about machine learning in digital hospitality.
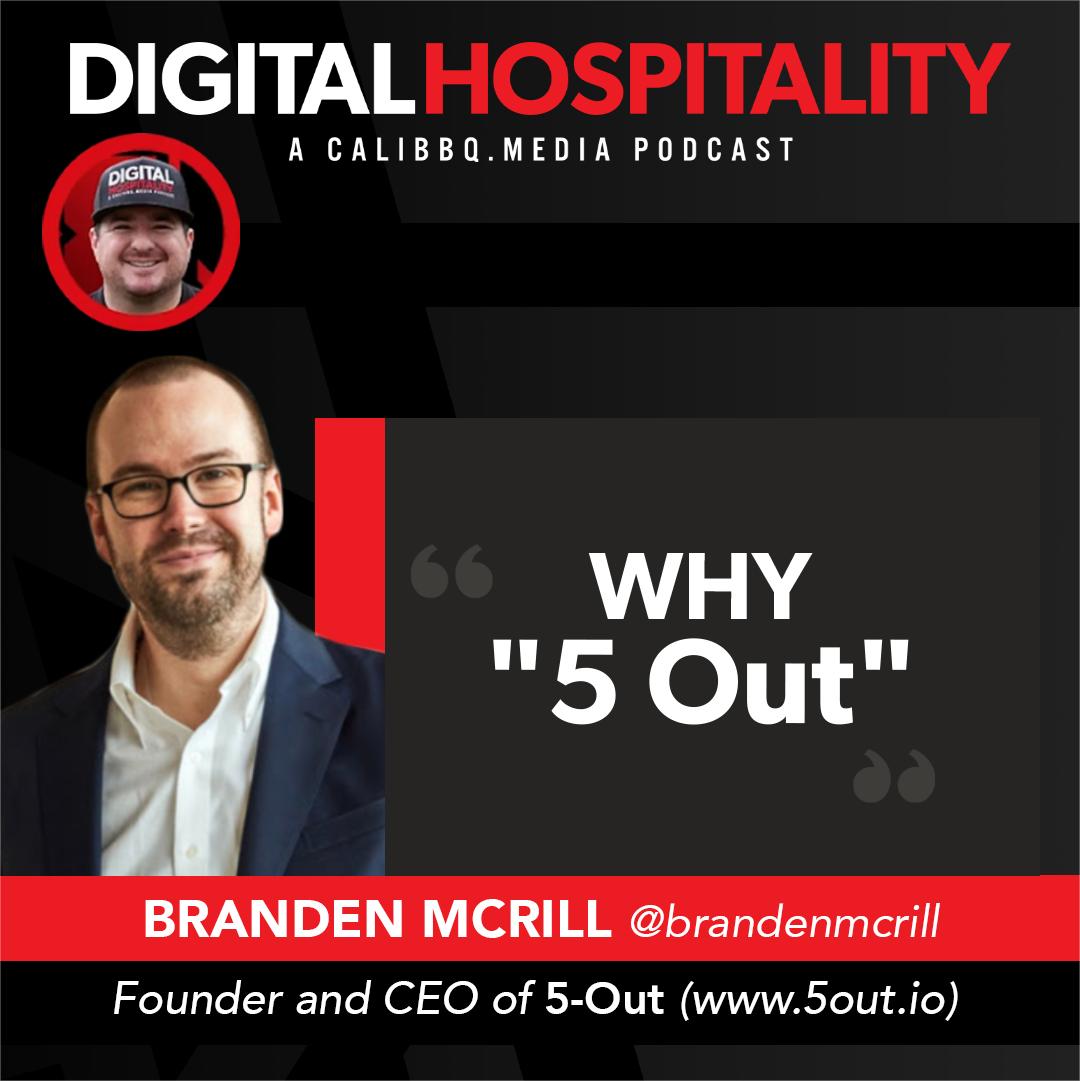
Branden in the Digital Hospitality Industry
Co-founder and CEO of 5 Out, Branden McRill wants to increase restaurant profitability by using data and machine learning to forecast performance. As an experienced restaurateur, McRill identified some improvement in the market and was inspired to found 5-Out as a means to use technology to improve digital hospitality.
This interview by Cali BBQ Media, invited Branden to share his story, who is the founder of 5-Out, a company that leverages machine learning to optimize restaurant operations. Branden explains the difference between machine learning and AI, the importance of machine learning for restaurant operations, and how his company collects and analyzes data to provide insights for restaurant owners. He also touches upon the challenges faced by the restaurant industry and how technology can help improve their profitability.
Machine Learning
The difference between Machine Learning and AI
Machine learning is a derivation of artificial intelligence. Machine learning is essentially a computer recognizing patterns through the absorption and digestion of data and with the understanding of those patterns. It then anticipates a likely and ultimately more continually likely probability of the next thing that will occur. So more simply said if I see something trending in a particular direction I can safely assume that that thing will continue to go in that direction unless there is some other indicator that comes in that says it won't and vice versa something is trending down. If there is no indicator that shows that it's going to be trending up then there will be no uptick.
Why Machine Learning is important for restaurant operations
There is a difference between a forecast and a projection. If you look at the textbook definitions of projections versus a forecast, the forecast is the thing that is likely to occur while the projection is the thing that you want to occur. One is subjective, other is objective.
Machine-learning model doesn't feel one way or another about any of the factors that are happening in your business currently and in that, it provides a great Voice of Truth that you can either follow or listen to or just consider, and it really up to you.
And that's the thing that I think is also really important to bring up on this call AIML (Artificial Intelligence Markup Language). It's for you to determine what percentage of the information that you're getting that you put into your decision-making process. That's the thing that people in the restaurant industry in particular will need to adopt as a part of their understanding of the process before artificial intelligence and machine learning make their way into the restaurant industry.
The difference between Machine Learning and Algorithms
The algorithm or algorithms multiple feed the machine learning. In other words, the machine learning allows the algorithm to grow.
Example: TikTok
So that's the same with social when I'm clicking on TikTok on certain content it's going to feed me through machine learning through the algorithm to make more content like that that I want to see.
Another Example: 5-Out
Another example is 5-Out. Each restaurant that we currently have a relationship with has an individualistic unique machine learning model that from day one is set to a particular starting point. And then we absorb up to three years of data currently or as little as four or five weeks if you're starting out. From the day that the machine starts digesting your sales data and your location data and your weather data and your scheduling data and your inventory management data and anything else that we can pull into the system. At a base minimum it's about 6,000 inputs coming per user.
How 5-Out Works
Where does the data come from and How it helps
Take the weather as an example. We pull that from a unique source which is a third party that we're partnered with to go 40 years back geotagged to a single block. And then we also use a separate tool that actually artificially utilizes its own artificial intelligence model to forecast 16 days forward. With that blending those two together in a ratio that makes the most amount of sense depending on what part of the country you are in what city you are in and also whether or not your business is increased or decreased by weather.
If you're in if there's a major Metropolitan City and people stay inside the building where they work. When it rains and they pummel your business because they don't want to walk outside so you might see an uptick when it rains. Machine learning will study your model to understand what was correlating and happening with the rain or not whatever weather is happening during that day and then make assumptions forward things.
If Valentine's Day is a Friday night you're going to see an uptick in business on Wednesday and Thursday for the people that don't want to go out on Friday. And in a business and then up to capture people who want to go on Saturday and Sunday. So there are so many dynamic points that this machine learning model actually ends up digesting as I said minimally digested about half a dozen in restaurants that have more data points that we're able to integrate with, sometimes it's over a dozen. But then it does multiple millions of variable calculations to come up with what it thinks is the most likely outcome of all that data. And then that day occurs and then it recollects the outcome. Because machine learning just like a human being doesn't like to be wrong, it then re-teaches and retrains itself to be more accurate the next day and the next day. So, the more data, the better, and the accuracy always increase over time.
Identify the Problem and Found 5-Out
The people in restaurants don't want to sit on a clock in an empty restaurant where they're not able to provide value. So what we are looking to do is to optimize outcomes for restaurant staff and teams as well as ownership by way of right-sizing the labor as well as the clocks. And the ultimate end goal here is we increase the net profitability of the business. So we're going backward our goal is for restaurant margins to have an infinitesimally small margin which is unfortunate because restaurants are also the largest labor for the course in the United States of America. So here are the largest workforce in the United States and to be able to take care of those people means that these businesses need to be successful. For them to be successful means that the small margins that they make already need to be as efficient as possible so that they can grow the businesses which then allows us to grow all the people that work in these restaurants.
If a business is going under people are going to be affected by way of loss of shifts may be potential loss of jobs decreased earnings and of course, that's not great. So what do we do we take the whole set of data that we can get for a single restaurant and we utilize that to forecast what's going to happen forward currently up to 35 days because many restaurants work on a four-four-five accounting schedule. This allows people to understand first and foremost what their revenues are going to be in advance. And then we ask them for their custom costs of goods and custom cost of Labor targets for their business model. We program those into the system and out comes a Labor budget that is either a percentage of revenue or is sales per labor hour or it can be a labor matrix if you know you want to have this amount of people working per this amount of people coming into the restaurant whatever it is. With that, you then get a weekly, daily, and hourly schedule and then you get a weekly and hourly clock budget with which to spend against. The whole goal is if I have 50 people coming in on a Monday night and I know I need x amount of people to come into staff. I know that they're going to order these things and I want to have that exact right amount because that's going to make the smoothest service so that guests are going to be happy and staff is going to be happy. Everything works out from a financial perspective in the end as a byproduct of purchasing on costs or under purchasing, or over-scheduling or under scheduling are the two greatest flights to the restaurant industry currently. Anything that can resolve and help provide visibility where there currently isn't in this crazy world that we live in.
We're trying to serve you by collecting this information on you so that you know who is coming in tonight, where they work, what they love, where they go to school, what are their interests and etc., so we can do something special and unique for them to surprise them.
Start a Restaurant Is Harder than Start a tech company
If I'm being very honest, I want to say that starting a restaurant is harder because of the multitude of variables uncontrollable. That's simply having to do with the nature of Real Estate Investors lenders, and the department of health, getting your guests turned on electrical, staffing, and all those things come to the starting point at one point. I would say that is more challenging and requires more juggling and that is an all-encompassing exhaustive job so you're really working.
In the capacity of starting a tech company, you have much more of a singular focus and there are fewer plates to spin. Perhaps some of those are a little bit more challenging but the success percentages I think are about the same. There is an equivalent difficulty in each but I have opened over 20 restaurants in my lifetime and I've only been materially part of this Tech startup I've been an advisor to some others and so I've seen the lens through some other people's eyes but I want to say restaurants are just the tiniest bit harder.
Share More Stories on Digital Hospitality
We originally set out to do 5-Out to take all the data that restaurants were sitting on and this was in 2016. I and my co-founder Mike originally got going on the just kind of conception of what this was going to become at the time point of sale reservations, inventory management, analytics systems but each one siled not connected. So you had to pull the data out to drop it into an Excel document and then pull in other data and then Mike who has been coding since he was 12 can do this very easily by writing some algorithms. we took this information from reservations and point of sale and inventory management and analytics and said okay let's drop all this information into this manual algorithm that Mike wrote and utilized to forecast what was going to happen because of all of the just automated generated reports. It's a ton of reading and then if you get into the customization reports of each of these systems you could sit around and do nothing else.
The underlying simple way to explain machine learning we have a bunch of different factors but each factor has a different percentage of importance and that changes and that are machine learning that's a really simple way to explain it so quoting a weight and a bias to each one of these different data points that's coming in from each one of these different silos and then utilizing that to forecast what's going to occur are even not enough. So forecasting revenue for us is just the very tip of the iceberg the next thing we do is automate the budget of labor and automate the budget of clocks to the point where even in the last two or three weeks we have actually launched the forecasting of item level sales. So forget here's how much money you need to spend this is actually what you're going to sell by the day by individual unit with this you can then automatically build purchase orders and prep lists that actually full transparency was what the original tool was intended to do full circle it was originally a company was called closed loop we actually changed the name to five out because we thought it was going to be a little bit more approachable.
Why 5-Out
We predict you know five minutes five hours five days and currently five weeks out so you have that visibility looking outwards but originally the company's name was Closed Loop. The whole concept of a Closed Loop is that you never actually have to get involved in the process. So what our next phase of the company is if we can automate your labor schedule and then we can understand through a relationship with seven shifts or a relationship with some other tool that's out there what the HR requirements are for people in terms of when do I want to work when can I work when am I working most efficiently when am I going to make the most amount of money and tie those two things together we can actually automate a schedule and then since we're all since we're already forecasting item level sales and also understanding what the demand is going to be and we know what your budget is then we can actually get to a point where we can automate prep lists we can automate purchase orders and in partnering with tools like Restaurant 365 and Craftable. we can then build purchase orders and prep lists which you then send directly to vendors for the restaurant tour.
We've started to help some of our restaurant clients and restaurant friends and guests who actually own other Hospitality businesses such as online stores, and retail shops. If you purchase new scheduled labor and you're in the hospitality industry, you can request help from us! We're starting to deal with some of those edge cases and work on some of those edge cases as well which is fun and interesting too.
Request a demo to utilize 5-Out to boost your restaurant's profit!